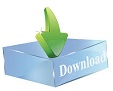

- PROGRAM SIMILAR TO METAMOVIE BUT FOR SONGS HOW TO
- PROGRAM SIMILAR TO METAMOVIE BUT FOR SONGS MOVIE
- PROGRAM SIMILAR TO METAMOVIE BUT FOR SONGS INSTALL
- PROGRAM SIMILAR TO METAMOVIE BUT FOR SONGS CODE
Movies_df = movies_df.apply(literal_eval) Let’s apply the literal_eval() function to the features.
PROGRAM SIMILAR TO METAMOVIE BUT FOR SONGS MOVIE
The movie data is present in the form of lists containing strings, we need to convert the data into a safe and usable structure. It should also include movies with the cast of the given query movie.įor that, we utilize the following features to personalize the recommendation: cast, crew, keywords, genres. Then the predictions should also include movies directed by the director of the film. Let’s say the query to our movie recommendation engine is “The Dark Knight Rises”. The accuracy of predictions made by the recommendation system can be personalized using the “plot/description” of the movie.īut the quality of suggestions can be further improved using the metadata of movie. Step 2: Build the Movie Recommender System Our new dataframe would be: movies_df.head() Movies_df = movies_df.merge(credits_df, on="id") Let’s merge the dataframes into one on the column ‘id’. We only need the id, title, cast, and crew columns of the credits dataframe. Movies_df = pd.read_csv(path + "/tmdb_movies.csv") Path = "./Desktop/TechVidvan/movie_recommendation"Ĭredits_df = pd.read_csv(path + "/tmdb_credits.csv") Let’s load the movie dataset using pandas. The credits file contains all the metadata information about the movie and the movie file contains the information like name and id of the movie, budget, languages in the movie that has been released, etc. The dataset contains two CSV files, credits, and movies. Step 1: Perform Exploratory Data Analysis (EDA) on the data
PROGRAM SIMILAR TO METAMOVIE BUT FOR SONGS HOW TO
How to build a Movie Recommendation System using Machine Learning In this project, we are building a Content-based recommendation engine for movies. For this system, metadata of the item is not required. Collaboration-based Filtering: These systems make recommendations by grouping the users with similar interests.Here, the main idea is if a user likes an item, then the user will also like items similar to it. Content-based Filtering: These suggest recommendations based on the item metadata (movie, product, song, etc).These types of systems are behind sections like “Top Trending”. Demographic Filtering: The recommendations are the same for every user.

There are 3 types of recommendation systems. Netflix, Amazon, and other companies use recommender systems to help their users find the right product or movie for them. These systems estimate the most likely product that consumers will buy and that they will be interested in. Recommendation systems are computer programs that suggest recommendations to users depending on a variety of criteria.
PROGRAM SIMILAR TO METAMOVIE BUT FOR SONGS CODE
Please download the python source code of movie recommendation system: Movie Recommendation System Project Code What is a Recommendation System?īefore moving on to build a recommender engine for movies, let’s discuss recommendation systems.
PROGRAM SIMILAR TO METAMOVIE BUT FOR SONGS INSTALL
pip install pandas scikit-learn Download Movie Recommendation System Project Code To install the above libraries on your machine, use the following command. Please download movie dataset: Movie Recommendation Dataset Tools and Libraries used But for this project, we are going to use a dataset that contains the metadata (cast, crew, budget, etc.) of the movie. There are several datasets available to build a movie recommendation system. In this machine learning project, we build a recommendation system from the ground up to suggest movies to the user based on his/her preferences. Keeping you updated with latest technology trends, Join TechVidvan on Telegram About Movie Recommendation Project
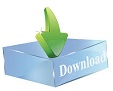